Discovering Textual Structures: Generative Grammar Induction using Template Trees
- Authors: Thomas Winters, Luc De Raedt
- Publication Date: 2020-09
- Publication Venue: Proceedings of the 11th International Conference on Computational Creativity
- Abstract: Natural language generation provides designers with methods for automatically generating text, e.g. for creating summaries, chatbots and game content. In practise, text generators are often either learned and hard to interpret, or created by hand using techniques such as grammars and templates. In this paper, we introduce a novel grammar induction algorithm for learning interpretable grammars for generative purposes, called Gitta. We also introduce the novel notion of template trees to discover latent templates in corpora to derive these generative grammars. By using existing human-created grammars, we found that the algorithm can reasonably approximate these grammars using only a few examples. These results indicate that Gitta could be used to automatically learn interpretable and easily modifiable grammars, and thus provide a stepping stone for human-machine co-creation of generative models.
Citation
APA
Winters, T., & De Raedt, L. (2020). Discovering Textual Structures: Generative Grammar Induction using Template Trees. Proceedings of the 11th International Conference on Computational Creativity, 177–180.
Harvard
Winters, T. and De Raedt, L. (2020) “Discovering Textual Structures: Generative Grammar Induction using Template Trees,” Proceedings of the 11th International Conference on Computational Creativity, pp. 177–180.
Vancouver
1.
Winters T, De Raedt L. Discovering Textual Structures: Generative Grammar Induction using Template Trees. Proceedings of the 11th International Conference on Computational Creativity. 2020;177–80.
BibTeX
Related talks
Related project
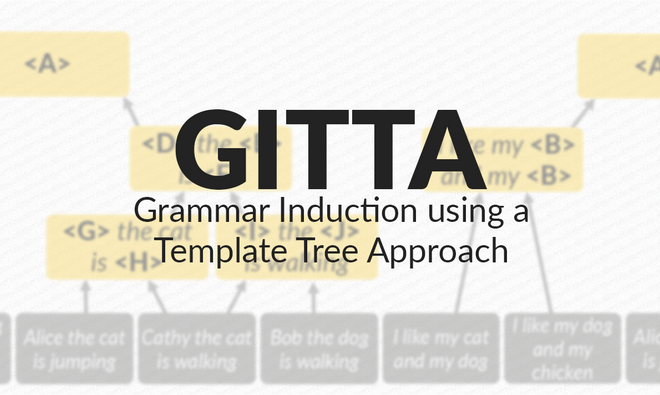
GITTA
Discovering textual structures to create generators